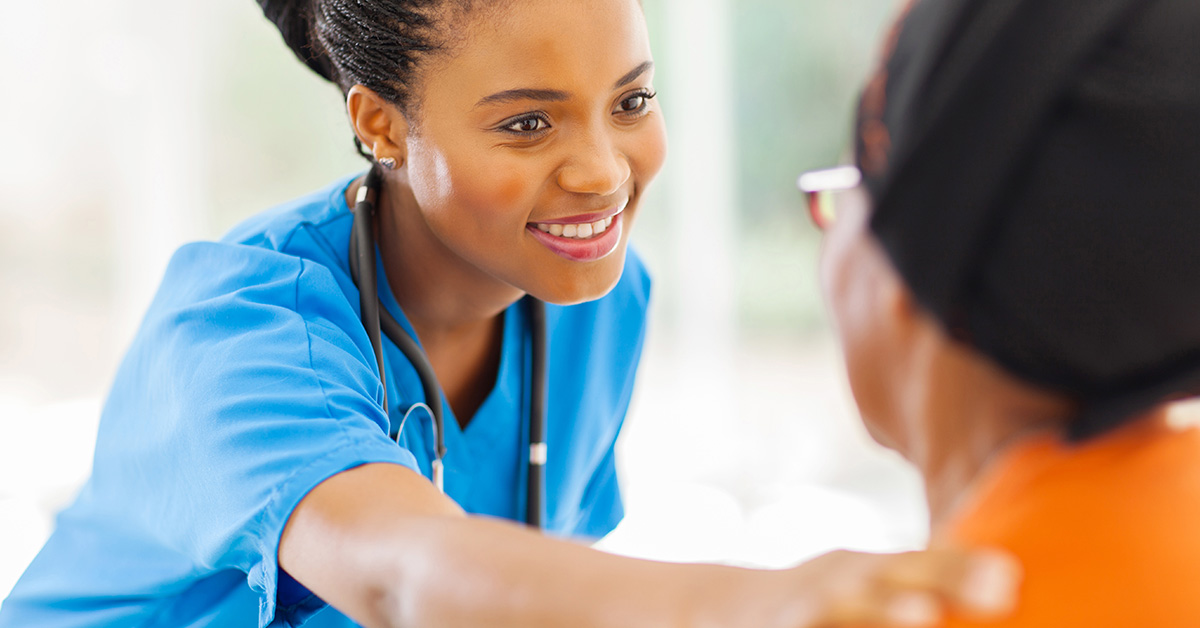
Using AI to improve cardiac strain analysis in the assessment of patients with heart failure
- | By Ultromics
- Strain, Articles, Heart Failure
The burden of heart failure (HF) on patients and clinicians, and the need to diagnose it more quickly and efficiently, is severe and constantly increasing. According to data from the Global Health Data Exchange registry, there were 64.3 million cases of heart failure in 2020 [1]. In the US alone, 8 million people are expected to suffer from the disease by 2030 [2], an increase of 2 million from what’s currently recorded [3].
Those patients experience debilitating symptoms and frequent hospitalizations, meaning HF can significantly impair quality of life. It also presents healthcare systems with substantial costs. In 2012, it was responsible for an estimated annual health expenditure of $31bn, more than 10 percent of the US cardiovascular disease budget, and is predicted to more than double over just two decades, to cost $69bn in 2030 [4].
Meanwhile, cardiologists, imaging technicians and clinicians face this rising workload already under pressure. A 2020 survey by the American College of Cardiology found that 35 percent of US cardiologists described themselves as “burned out” [5]. But there is a way of significantly improving outcomes for both patients and practitioners alike.
In echocardiography - the most popular modality used to diagnose heart failure - artificial intelligence (AI) can analyze global longitudinal strain (GLS) which is proven to be a better and earlier indicator of heart failure as it picks up preserved ejection fraction (HFpEF). Used in tandem with ejection fraction analysis, GLS analysis via AI increases diagnostic speed and quality. This analysis, as carried out by Ultromics’ EchoGo, uses AI that is 100 percent precise all the time, every time.
The case for using Global Longitudinal Strain (GLS) analysis
GLS analysis is not as widely used or accepted as EF, not least because some see strain analysis as too expensive and time-consuming. However, the European Society of Cardiologists (ESC) recommends the use of global longitudinal strain as a predictor of heart failure, noting that strain can detect patients with preserved ejection fraction [6].
The role of GLS in predicting heart failure severity was highlighted in a study of almost 2,200 patients, published in February 2021 [7]. The observational, prospective cohort study looked at the GLS of 2,186 people with stages A-to-D heart failure according to the American Heart Association (AHA) criteria. The team found that GLS was not only associated with HF severity, but during a median follow-up period of just over three years, was predictive of all-cause mortality and cardiac death. It confirmed the conclusion of a 2019 review of the evidence, which found GLS had “greater prognostic value” than Left Ventricular Strain (LVEF). “GLS adds incremental value to EF in the prediction of adverse outcomes.”[8]
Comparisons with manual analysis of echocardiograms have shown GLS analysis using AI minimizes variability and can more accurately predict a patient’s known outcome, where commonly used echocardiographic methods are complex and dependent on multiple measurements and operator experience and thus can have lower accuracy.
Operator variability can impact diagnostic accuracy and, currently, software for interpreting ECG scans is only semi-automated and requires partial manual tracing of endocardial borders which is operator dependent and creates high levels of inter- and intra-operator variability [9]. Eliminating variability would deliver consistent, reliable scans, and improve disease detection and operational efficiency. Some studies of manual analysis have shown up to 10 percent variability, where as Ultromics’ EchoGo has zero variability [10].
GLS analysis with AI
When using EchoGo for GLS analysis with AI, the automated process means that the same quality of care can come from all levels of clinician, including trainees. The zero variability guaranteed by the AI powering EchoGo ensures standardized accurate results, which are provided in an eight-page report delivered, on average, 20 percent quicker than a report derived with manual input using non-AI software [11]. It fully automates over 230 measurements, including strain, left ventricular volumes and ejection fraction, for more consistent, timely heart disease detection.
EchoGo reports are sent without ‘hands-on software’ time, eliminating any subjectivity, because EchoGo operates in the cloud – there is no software to download, and no need to upgrade hardware. And since it is in the cloud, analysis can also be provided wherever cardiology is practiced, from the smallest imaging center to the largest hospital.
GLS via AI can improve the quality of heart failure diagnosis, saving time for clinicians while increasing accuracy of results and satisfaction for patients, who get the same level of care no matter who they see. It can also lower costs too, by reducing wait times and instances of readmission. This advantage is not a future tool: it is available now, simply and cost-effectively implemented as a vendor-neutral solution into any cardiology set-up, in the form of EchoGo.
References:
- Lippi G, Sanchis-Gomar F. Global epidemiology and future trends of heart failure. AME Medical Journal. 2020 Jun;5:15–5.
- Savarese G and Lund LH. Global public health burden of heart failure. Cardiac Failure Review. 2017;3:1:7-11.
- Virani SS, Alonso A, Aparicio HJ, et al. Heart Disease and Stroke Statistics—2021 Update: A Report From the American Heart Association. Circulation. 2021;143(8).
- Savarese G and Lund LH. Global public health burden of heart failure. Cardiac Failure Review. 2017;3:1:7-11.
- Women, Mid-Career Cardiologists Most Likely to Report Symptoms of Burnout [Internet]. American College of Cardiology. 2020
- Ponikowski P, Voors AA, Anker SD, et al. 2016 ESC Guidelines for the diagnosis and treatment of acute and chronic heart failure. European Heart Journal. 2016;37:2129–200.
- Tröbs S, Prochaska JH, Schwuchow-Thonke S, et al. Association of Global Longitudinal Strain With Clinical Status and Mortality in Patients With Chronic Heart Failure. JAMA Cardiology. 2021;6:448–456.
- Ashish K, Faisaluddin M, Bandyopadhyay D, Hajra A, et al. Prognostic value of global longitudinal strain in heart failure subjects: A recent prototype. IJC Heart & Vasculature. 2019 Mar;22:48–9.
- Fully automated quantification of contrast and non-contrast echocardiograms eliminates inter-operator variability. [Internet]. www.ultromics.com. 2020