Using AI to make precision heart failure detection possible
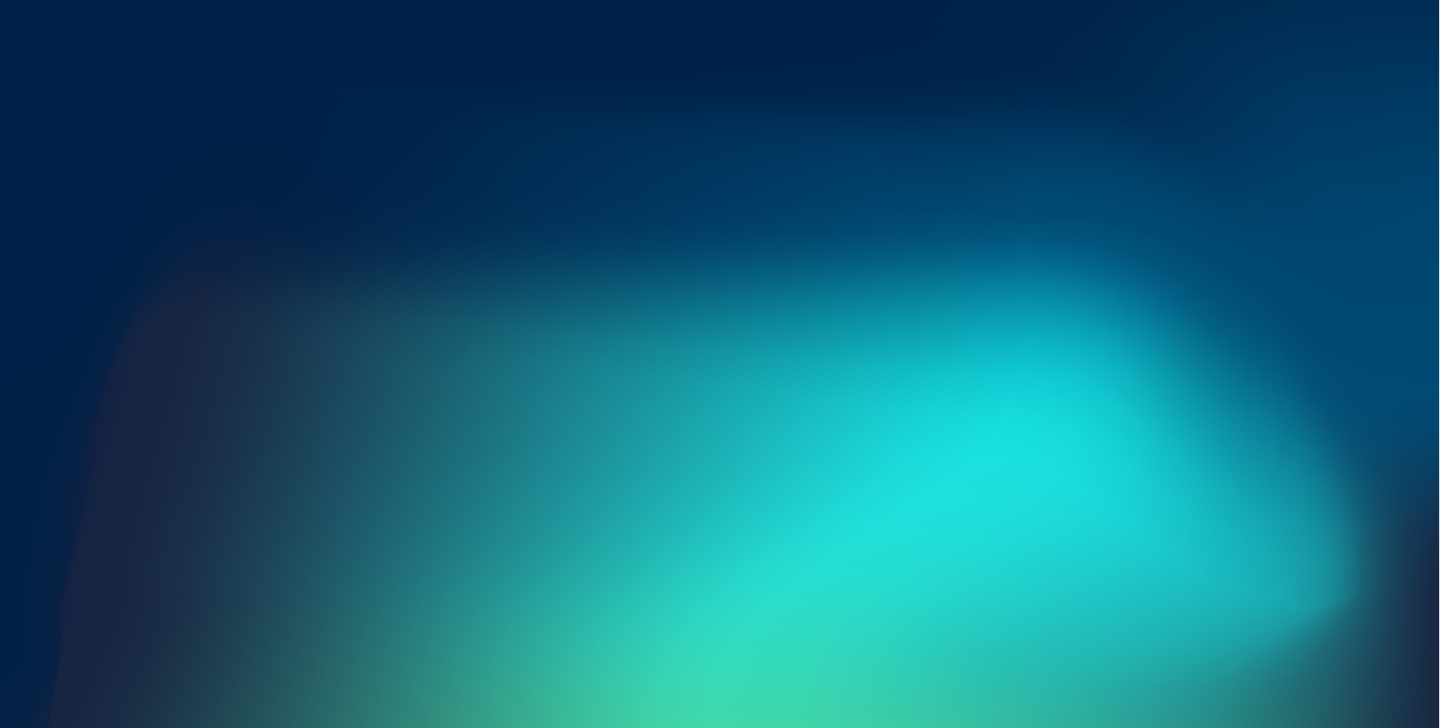
Up to 75% of heart failure patients are missed. 1
High rates in missed cases are associated with an increase in hospitalizations, high readmission rates, unnecessary procedures, and often death.
To improve and extend lives, and decrease associated costs, we need to detect heart failure earlier and more accurately. This is especially true for patients with heart failure with preserved ejection fraction (HFpEF).
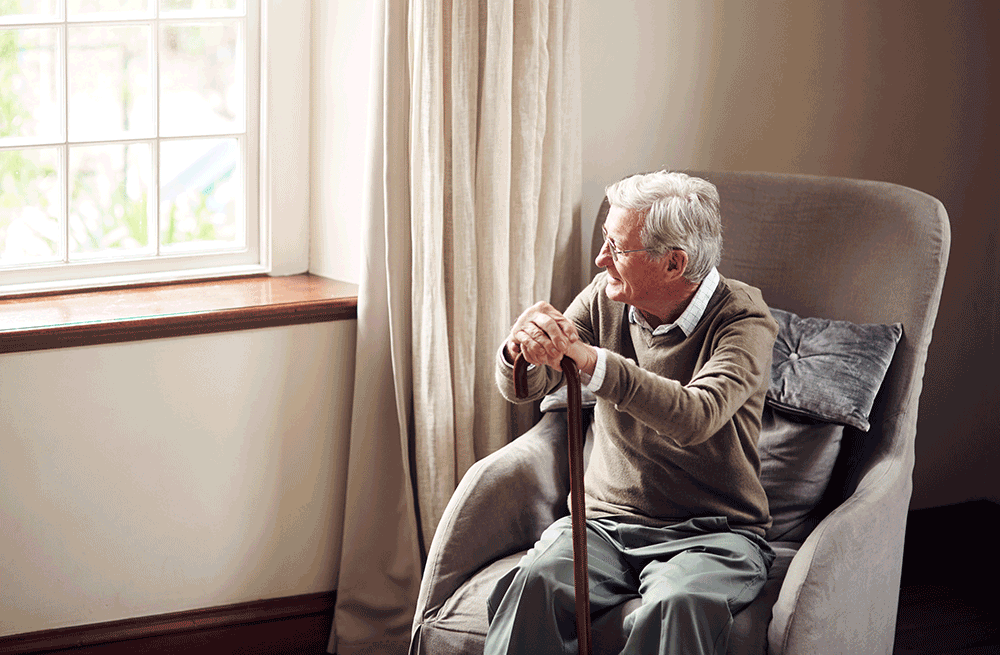
Precision heart failure detection
Ultromics combines echocardiography - the most common and cost-effective cardiac imaging modality - with AI-derived insights that go beyond what the human eye can detect.
Ultromics’ AI specializes in early and accurate heart failure detection and risk stratification, including HFpEF, to reduce hospitalizations and help improve patient outcomes.
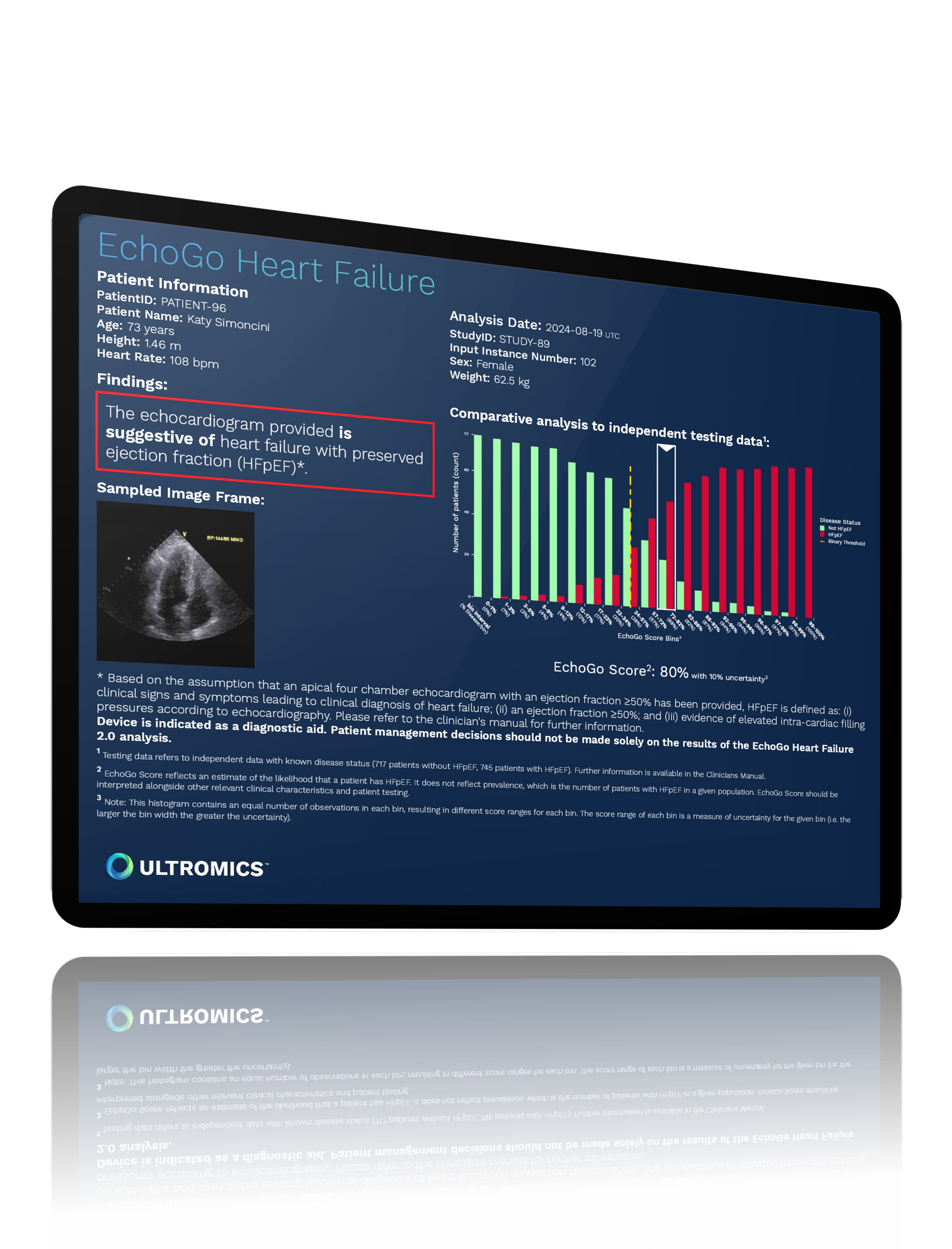
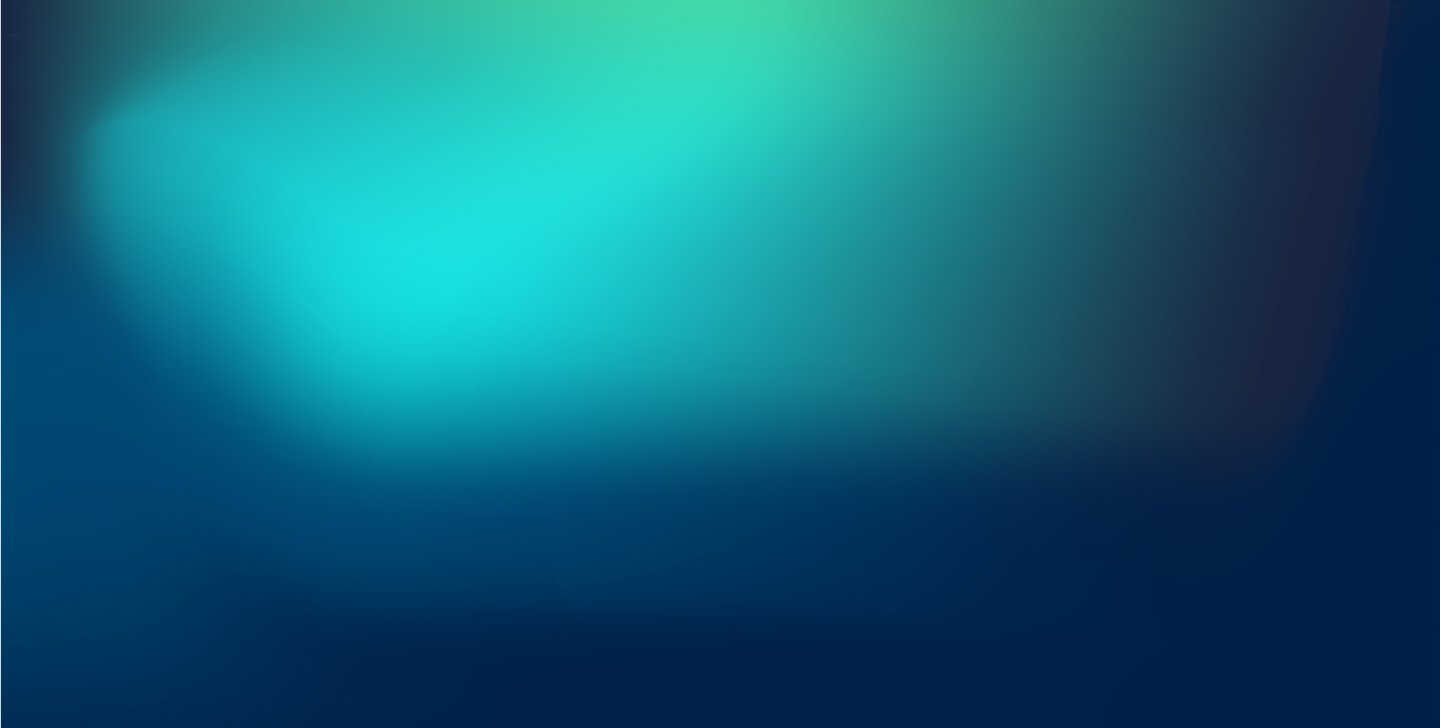
The need to improve heart failure costs is critical
$25,000
The annual median medical costs for heart failure care per patient.2
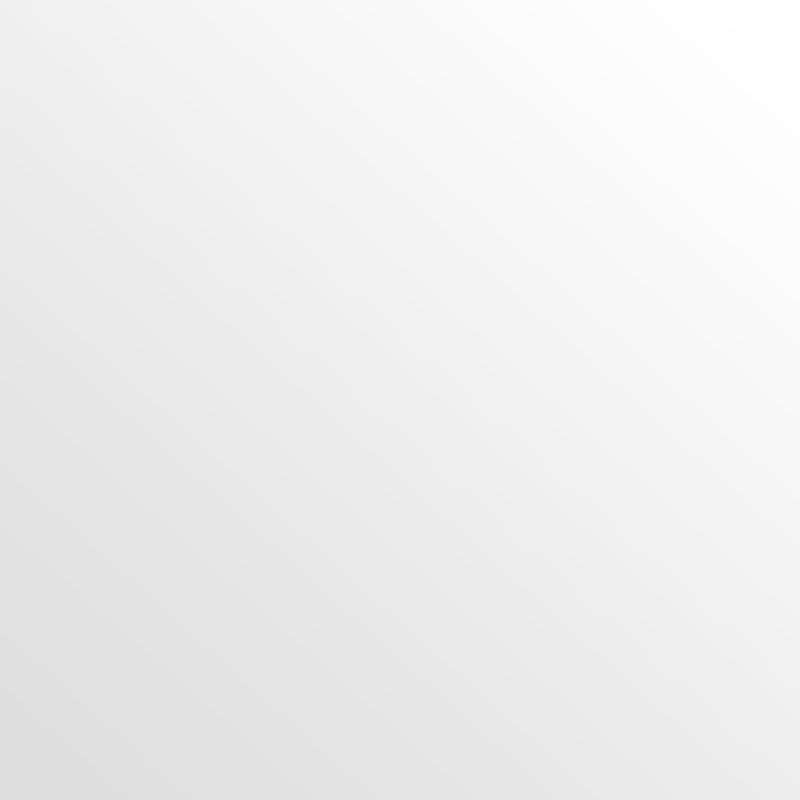
80%
The number of direct heart failure costs attributed to inpatient stays.
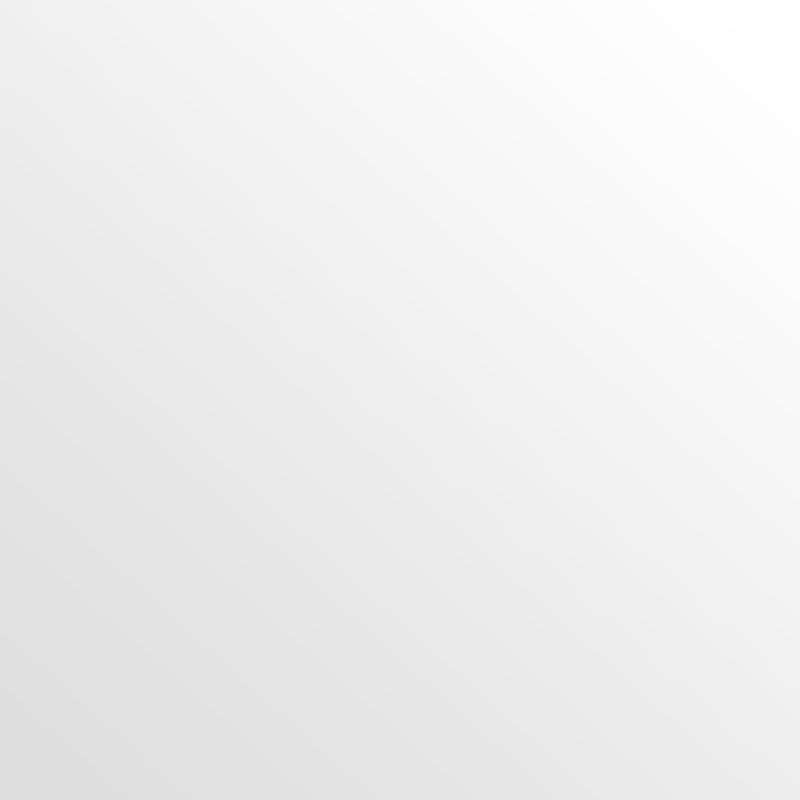
> 3 million
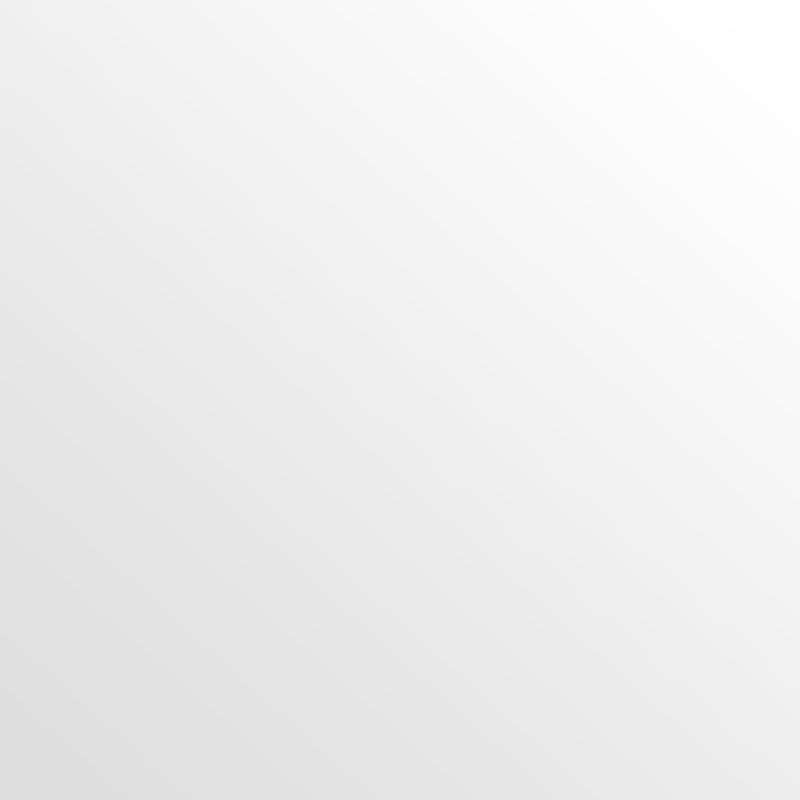
#1 driver
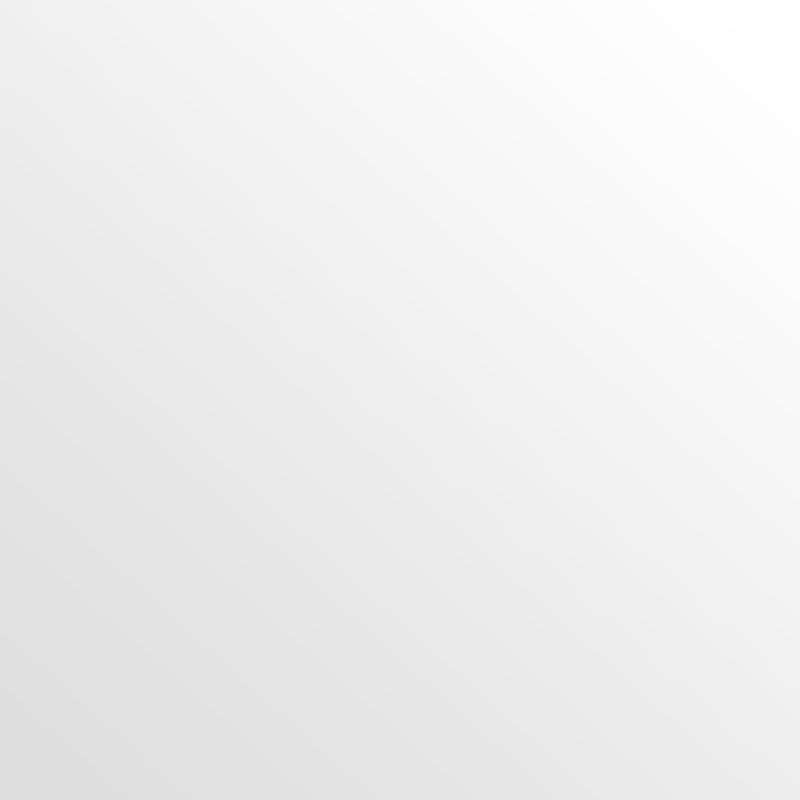
Health Insurance providers play a pivotal role in addressing the expanding heart failure crisis
More focus needed on HFpEF
Heart failure with preserved ejection fraction (HFpEF) is the single greatest unmet need in cardiovascular medicine. [3]
HFpEF is more costly than the more commonly diagnosed heart failure with reduced ejection fraction (HFrEF) and represents a greater proportion of heart failure costs. [4]
HFpEF diagnosis is complex and replies on often inconclusive diagnostic algorithms and invasive testing – such as catheterization – which contributes to it being missed up to 75% of the time. [1]
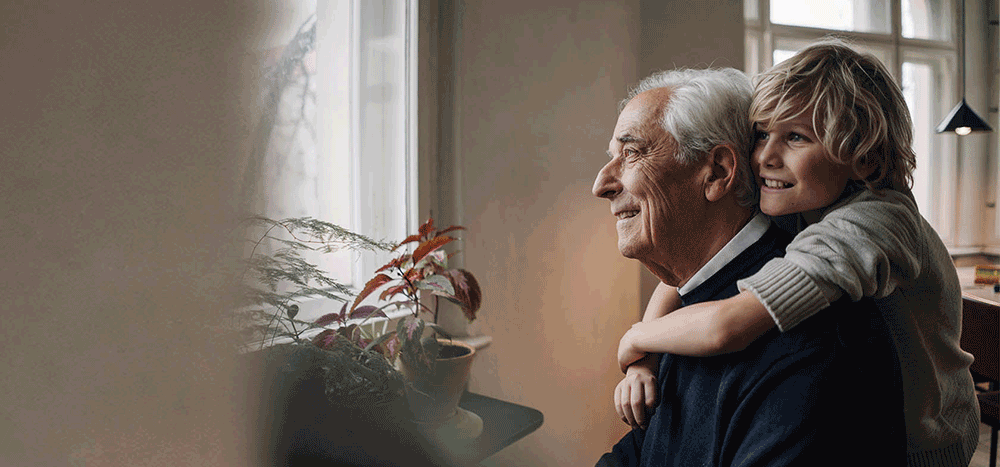
Why precision matters
New treatments make it more important than ever to quickly and accurately diagnose heart failure
Sodium-glucose cotransporter 2 inhibitors (SGLT2is) and other new drug therapies have been shown to improve both HFrEF and HFpEF patient outcomes. [6]
These SGLT2is can reduce death and hospitalizations by 20-40%. [5]
Effective and fast diagnoses lead to earlier treatment with these new classes of drugs, and can have a significant outcome when combined with effective patient management.
Echocardiography remains the safest and most accessible option
Echocardiography is already the most common means of diagnosing heart failure, with over 30 million exams being performed each year. [7]
Echocardiography is low-cost, accessible, portable, and safe for patients, and with AI automating insights, it removes manual time, subjectivity and variability in calculating analysis, and provides accurate heart failure diagnostics to any care setting.
The benefits of AI for payers
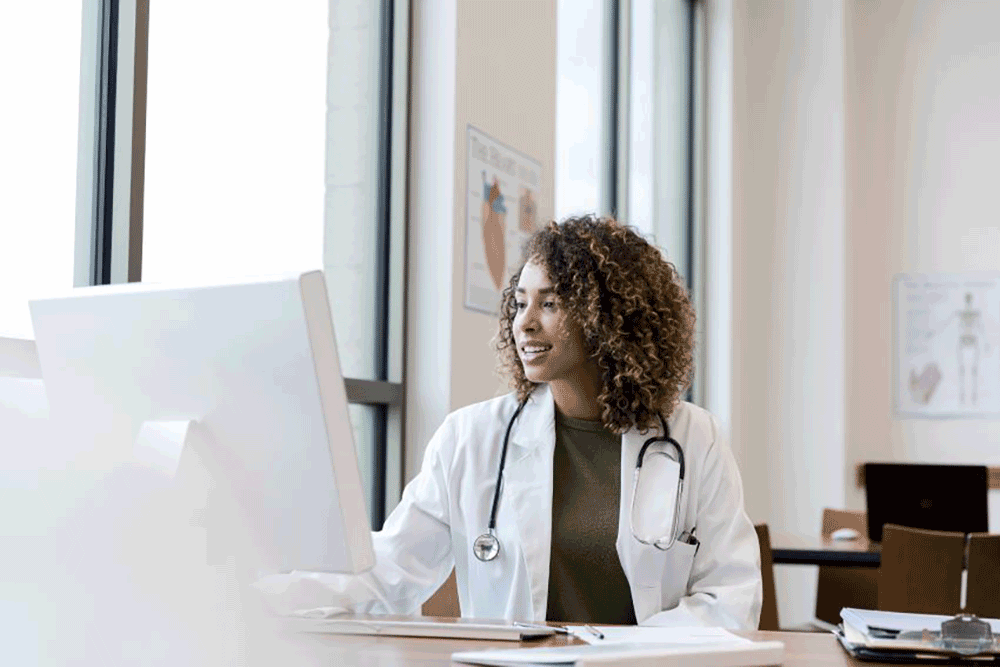
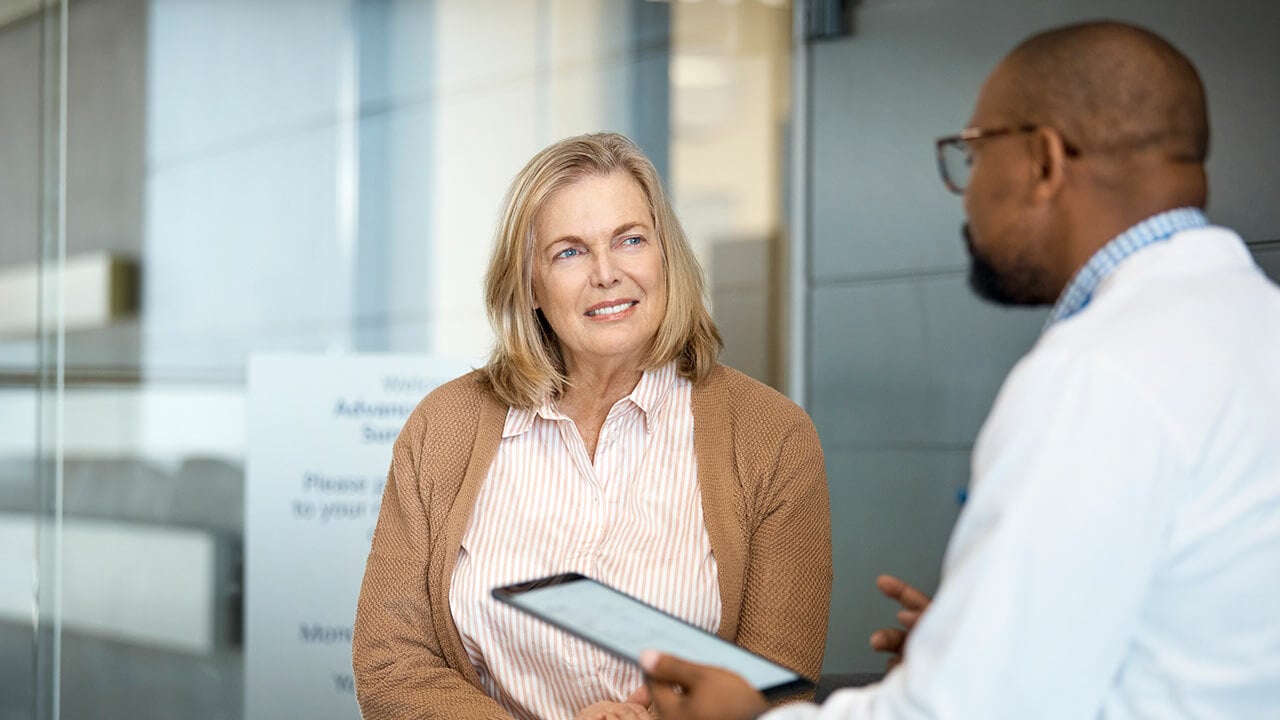
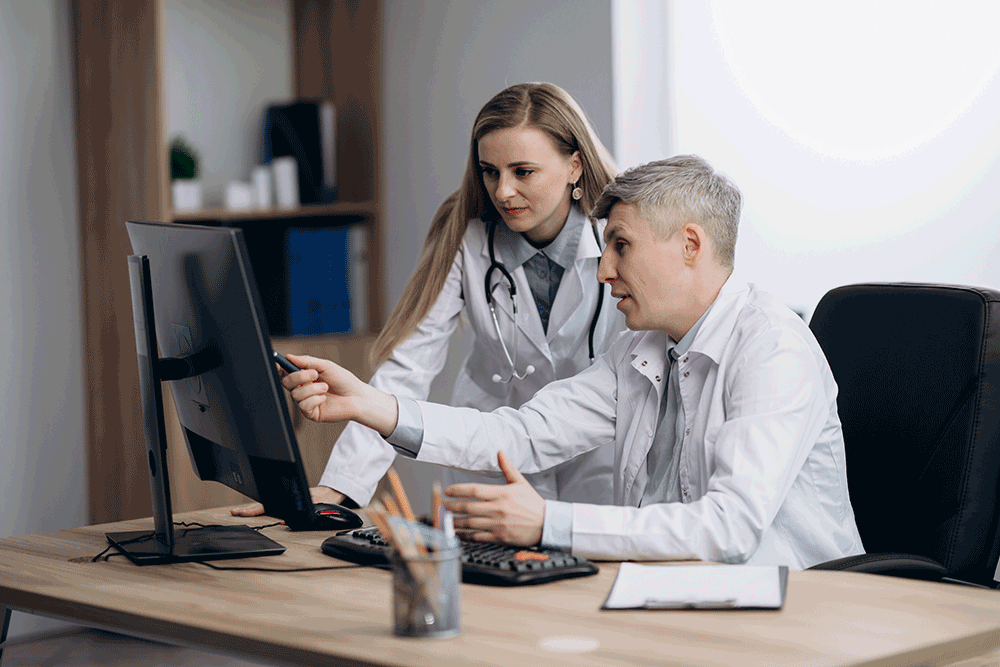
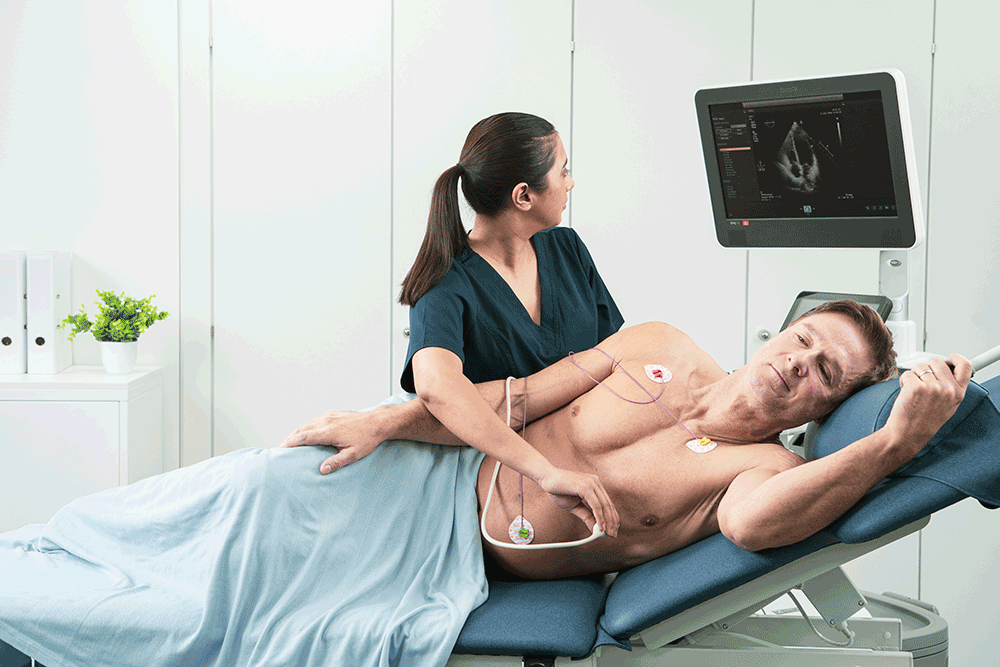
AI-driven insights consistently deliver accurate, reproducible results that reduce the costs associated with rehospitalization and invasive procedures.
Ensure accurate detection at the time of care, reducing waste and over-treatment, with AI built on ten years of known patient outcomes.
AI automation enables effective heart failure detection and risk stratification at a population level.
AI quantifies echocardiographic measurements, levelling up echo as a more effective tool for detecting and risk-stratifying heart failure.
References:
- Sanders-van Wijk S, Aizpurua AB, Brunner-La Rocca HP, et al. The HFA-PEFF and H2FPEF scores largely disagree in classifying patients with suspected heart failure with preserved ejection fraction. European Journal of Heart Failure. 2021;23:838-840
- Urbich M. Globe G, Pantiri K, et al. A Systematic Review of Medical Costs Associated with Heart Failure in the USA (2014-2020). Pharmacoeconomics. 2020;38:1219-1236Shah S, et al. Circulation. 2020;141:1001–1026
- Nichols GA, et al. J Am Coll Cardiol. 2022;79: (9 Supplement):283
- Cunningham JW, Vaduganathan M, Claggett BL, et al. Dapagliflozin in Patients Recently Hospitalized With Heart Failure and Mildly Reduced or Preserved Ejection Fraction. Journal of the American College of Cardiology. 2022;80:1302–1310
- Heidenreich PA, Bozkurt B, Aguilar D, et al. 2022 AHA/ACC/HFSA Guideline for the Management of Heart Failure: A Report of the American College of Cardiology/American Heart Association Joint Committee on Clinical Practice Guidelines. Journal of the American College of Cardiology. 2022;79:263-421
- Deloittes Proprietary Data
Curious about upcoming research and innovation?
Sign up to hear about the latest news.