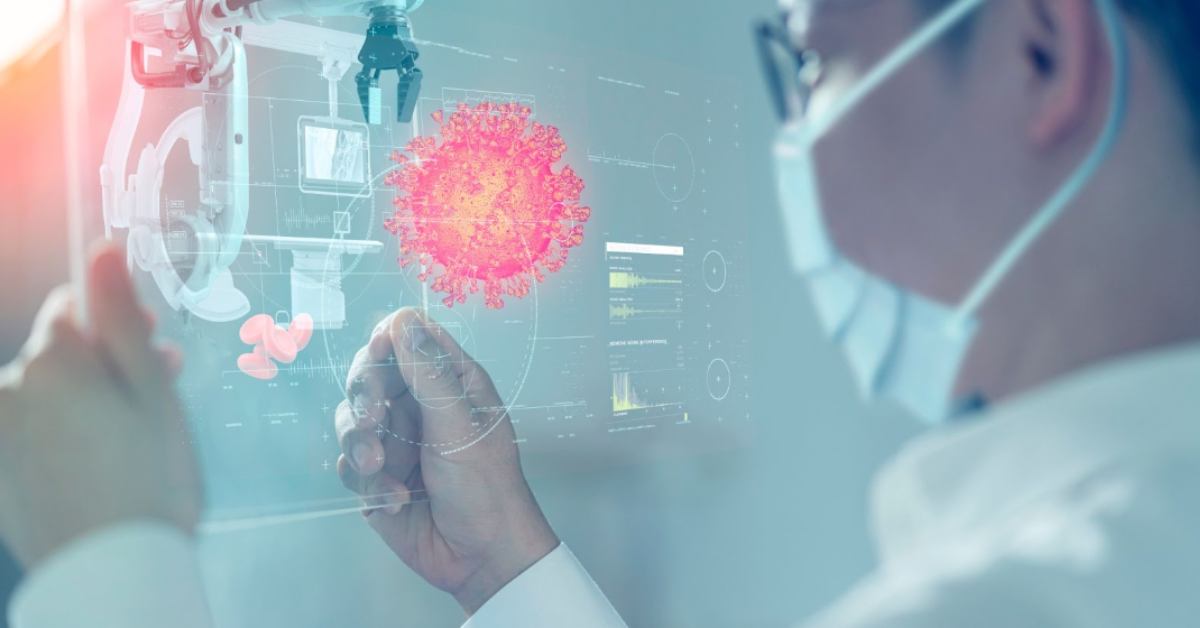
Achieving Greater Prognostic Power in Echocardiography: The Latest Myocardial Strain Research Using AI
- | By Ultromics
- Articles
Myocardial strain has become a key component for successfully diagnosing and risk stratifying heart failure (HF) patients, with the 2022 AHA/ACC/HFSA Guideline for the Management of Heart Failure now recommending the use of global longitudinal strain (GLS) in routine clinical workflow. [1]
In addition, the 2021 EACVI and ASE Guidelines for Non-invasive Imaging in Coronary Syndromes also recommend strain be measured on all echo tests, acknowledging the metric is more effective at detecting early changes in cardiac function for coronary syndromes, and predicting outcomes than the traditionally used ejection fraction (EF).
However, routinely adding GLS to ejection fraction can be difficult to manage. This is why innovative AI technologies are beneficial for clinicians looking to start or scale their strain program. Newer AI platforms, like Ultromics’ EchoGo, have been shown to automate strain parameters quickly and with zero variability, and can be added easily into routine workflow without additional time and effort, and with minimal cost, through a secure cloud-based subscription that provides a fully-managed LV analysis service.
Among the multitude of studies to date, there are two pieces of research which suggest the benefits of AI for efficiently and accurately measuring strain for CAD and heart failure.
The first study looks at using AI to measure left ventricular ejection fraction (LVEF) and global longitudinal strain (GLS), and it shows promising results for its ability to improve CAD sensitivity from 0.78 to 0.83.
The second study was completed during the COVID-19 pandemic and focused on using AI to measure strain, outperforming manual analysis at predicting death in patients with the disease.
Both of these studies showcase not only why it’s important to use strain in routine testing, but also how AI can streamline processes and improve accurate risk stratification.
Accuracy of AI-powered GLS to Detect Obstructive Coronary Artery Disease
Stress echocardiography (SE) is one of the most widely used procedures for both the diagnosis and risk stratification of patients with known or suspected coronary artery disease (CAD).
LV longitudinal strain measurements may be able to detect significant CAD in patients undergoing stress echocardiography and coronary angiography, who have normal resting wall motion.
In addition, while quantification of the left ventricle has been shown to improve diagnostic accuracy, this process generally requires manual contouring and/or offline analysis. The extra time needed to measure, coupled with inter- and intra-operator variability, can negatively impact patient outcomes.
To test how AI can solve these challenges, researchers sourced an international multi-center population of 500 patients with suspected CAD. They applied EchoGo’s AI pipeline, which is capable of fully automated contouring for LVEF and GLS analysis of both non-contrast and contrast SE images.
AI-derived contours of the endocardial border were automatically generated for apical 2- and 4-chamber views. End diastolic (ED) and end systolic (ES) frame selection, and consequently cycle selection, were automatically determined by the software based upon LV volume. Follow-up consisted of reviewing medical records for up to one year after the SE was obtained.
The study found that ischaemic patients had significantly greater AI-measures of LV volumes and significantly lower LVEF and GLS at rest and peak SE. Peak LVEF and peak GLS were significant independent predictors of CAD.
The important takeaway was that the addition of automated LVEF and GLS to basic models significantly improved the C statistic from 0.78 to 0.83 and 0.85 (both p<0.001), respectively.
The researchers concluded that fully automated LVEF and GLS in non-contrast and contrast SE images is not only feasible, but it can independently improve the prediction of obstructive CAD above and beyond traditional SE indexes. This significant piece of research showcases how AI can be instrumental in successfully predicting outcomes, especially for patients with obstructive CAD.
Read more about using AI to Measure LVEF and GLS for obstructive Coronary Artery Disease
Predicting Heart Failure with AI-powered GLS
The severe acute respiratory virus that led to the global COVID-19 pandemic is known to adversely affect the cardiovascular system through both direct and indirect mechanisms.
This is why the World Alliance Societies of Echocardiography (WASE) conducted an international multi-center study to determine the clinical and echocardiographic phenotype of acute cardiac disease in COVID-19 patients.
Using EchoGo Core, the researchers explored phenotypic differences in different geographic regions across the world. They also identified which echocardiographic parameters are most predictive of early clinical outcomes, such as heart failure.
The study consisted of 870 patients with acute COVID-19 infection from 13 medical centers located in Asia, Europe, the United States, and Latin America. These patients had undergone transthoracic echocardiograms (TTEs), and their clinical and laboratory data were collected, including patient outcomes during their initial hospitalization. Anonymized echocardiograms were then analysed with EchoGo Core to calculate left ventricular (LV) volumes, ejection fraction (EF), and LV GLS.
Among some of the key takeaways included markers that were independently associated with mortality: left ventricular longitudinal strain (LV LS), right ventricle free wall strain (RV FWS), age at presentation, lactic dehydrogenase (LDH), and previous lung disease. The study found that alternately, left ventricle ejection fraction (LVEF) was not independently associated with mortality in the patients analyzed.
Another important finding was that fully automated quantification of LVEF and LVLS using AI minimized variability. EchoGo’s powerful AI was able to not only accurately quantify these strains, but also predict in-hospital and follow-up mortality.
EchoGo’s AI was found to achieve more accurate predictions of these mortalities than manual interpretation. This indicates the power of AI in predicting patient outcomes with zero variability.
Read more about using AI to predict outcomes in COVID-19 patients
Adding strain to routine measurements is a recommended approach per new guidelines. However, the added time it takes to measure, coupled with operator variability, training, and potential software purchases, can make it difficult to implement strain in real-world settings.
With EchoGo’s fully automated zero variability measurements, healthcare providers can make efficient and informed decisions to improve patient outcomes.
References:
- Heidenreich PA, Bozkurt B, Aguilar D, et al. 2022 AHA/ACC/HFSA Guideline for the Management of Heart Failure: A Report of the American College of Cardiology/American Heart Association Joint Committee on Clinical Practice Guidelines. Journal of the American College of Cardiology. 2022;79:263-421.
- Fully automated left ventricular ejection fraction and global longitudinal strain predicts obstructive coronary artery disease in patients undergoing stress echocardiography: A multi-centre study. [Internet]. www.ultromics.com. 2021 [cited 2022 Jul 12].
- Karagodin I, Carvalho Singulane C, Woodward GM, Xie M, Tucay ES, Tude Rodrigues AC, et al. Echocardiographic Correlates of In-Hospital Death in Patients with Acute COVID-19 Infection: The World Alliance Societies of Echocardiography (WASE-COVID) Study. Journal of the American Society of Echocardiography. 2021;34(8):819–30.