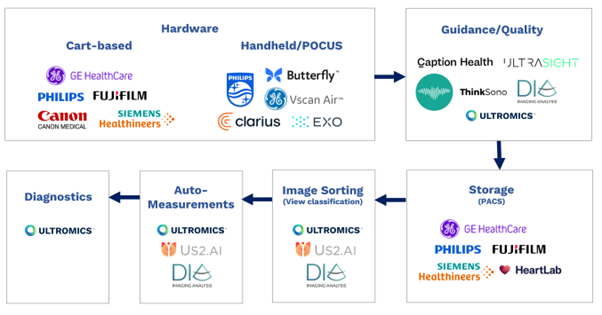
10 Years in the Making: The Clinical Application of AI-Based Echocardiography Diagnostics
- | By Ultromics
- Articles, Heart Failure
Dr Ross Upton PhD, Founder, CEO and Chief Scientific Officer, Ultromics, and AI Co-Chair, HeartShare, NIH
AI-based Echocardiography Diagnostics (AI EchoDx) has come a long way since I first started working on the approach at the University of Oxford in 2014. Countless papers have been published, numerous solutions have been developed, and, just in the last year, the Centers for Medicare and Medicaid Services (CMS) has assigned reimbursement codes.
The approach has gone from theory to real-life practice in a decade. Now, we hold in our hands the ability to significantly enhance the diagnostic capabilities of echocardiography so that people living with life-threatening cardiovascular conditions are no longer missed.
In this, the first in my series of monthly blogs, I want to bring you up to speed on the emerging field of AI EchoDx, the journey so far, outline the current landscape, and talk about what more needs to be done to bring this disruptive technology to the fore.
The Big Picture
From hardware and software, to storage, image sorting and auto-measurements, all elements of the echocardiography pipeline have been transformed by AI in recent years.
Cart-based hardware industry leaders Philips, GE Healthcare, Siemens, Fuji, and Cannon, and hand-held innovators such as Butterfly, Clarius, Exo, Philips Lumify, and GE Vscan are all now offering some form of AI. I recommend following Reza Zahiri’s LinkedIn for more in-depth analysis of the Ultrasound hardware market.
Companies such as Caption Health (recently acquired by GE), Ultrasight, ThinkSono provide AI-driven image acquisition guidance with Dia (recently acquired by Philips) and Ultromics having the capabilities to provide image quality feedback.
Storage, or picture archiving and communication systems (PACS), from major players including Philips, GE Healthcare, Siemens, Fuji, and Heartlab have been enhanced with AI, as have image sorting and view classification solutions. These are currently provided by companies including us at Ultromics, Dia, and US2.ai, who all also offer auto-measurements.
AI EchoDx is the last part of the jigsaw. And now, with the FDA clearing two of Ultromics’ modules, and the CMS assigning reimbursement codes to our applications, it’s time for the approach to take its place in clinical practice.
Echocardiography AI pipeline diagram
Evolving Landscape
Traditional echocardiography imaging is the bedrock of cardiovascular disease (CVD) diagnosis. But is also complex and time-consuming, requiring a highly specialized and increasingly rare skillset.
As such, diagnoses can be missed, leading to people not receiving the care they need. Heart failure with preserved ejection fraction (HFpEF), for example, can go undetected in up to 64% of cases.1 This can lead to adverse outcomes, up to and including preventable deaths.
While undertaking a PhD in cardiovascular medicine at the University of Oxford, I saw the potential for using AI to overcome some of these limitations – to develop a tool that could help clinicians make more accurate diagnoses.
With this goal in mind, myself Professor Paul Leeson, who co-founded Ultromics, designed our first AI EchoDx algorithm. The aim was to improve the detection of significant coronary artery disease (CAD) from a stress echocardiogram from 76% to over 90%. We designed the initial algorithm in 2014, and we filed our first patent in 2016.2
Of course, we were not the only ones working in this space. Two of my favourite papers from this time are by Sanjiv Shah et al, who looked at using unsupervised learning to determine novel phenotypes of HFpEF in 2015,3 and Partho Sengupta’s group, who published on using supervised machine learning to differentiate hypertrophic cardiomyopathy (HCM) from athlete’s heart in 2016.4
Phenomapping for Novel Classification of Heart Failure With Preserved Ejection Fraction on Circulation, Machine-Learning Algorithms to Automate Morphological and Functional Assessments on JACC Journals.
But Paul and I were the first to attempt to bring AI EchoDx to the clinic. Ultromics, the company we spun out of the university in 2017, launched the CAD algorithm as Echo Go Pro, and in 2020, (with the help of team Ultromics) it became the first solution of its kind to receive FDA clearance. Two years later, our paper was published in JACC Cardiovascular Imaging.5 The FDA has since approved our second AI EchoDx, for HFpEF, which was built in collaboration with Dr Patricia Pellikka from the Mayo Clinic and published in JACC Advances.
EchoGo Pro and EchoGo HFpEF remain the only FDA-cleared AI EchoDx modules. Last year, we also received FDA breakthrough designation for our third algorithm, EchoGo Amyloidosis. We have partnered with both JnJ Innovation and Pfizer to bring this module to market.
Automated Echocardiographic Detection of Severe Coronary Artery Disease and Heart Failure with Preserved EF Using Artificial Intelligence in JACC Imaging and JACC Advances, respectively.
Why create AI EchoDx for HFpEF and Amyloidosis? Well, because while treatment options for these conditions have been lacking in the past, both now have effective therapies that have been shown to reduce hospitalisation and mortality.7,8 However, both diseases are markedly under-diagnosed,1,9 meaning many people are never prescribed the medication they need.
The more accurate, more timely diagnosis they provide could help keep people out of hospital. And with heart failure (HF) hospitalizations predicted to cost the US $69bn a year by 2030,10 all stakeholders, from patients and clinicians to providers and insurers, can agree that this is a worthwhile goal.
Reimbursement Breakthrough
Ultromics is the only company with FDA-cleared AI EchoDx modules, and, I believe, the only echo company delivering our products through a SaaS model.
But importantly, we have also secured CMS reimbursement codes, a major milestone in making the leap into routine clinical care.
Previously, acquisition, storage, sorting and measurements were all bundled into one code, either for the full transthoracic echocardiogram (TTE), limited or follow-up TTE, or stress echo. It meant there was no additional reimbursement for using AI software that, for example, generated automated measurements (outside of strain), and limited return on investment for teams wanting to adopt the technology.
Last year, Ultromics was able to work with CMS to introduce two new echocardiography codes, specifically for AI EchoDx:
- Outpatient HCPCs code - C9786– $284.88 - Echocardiography image post-processing for computer aided detection of HFpEF, including interpretation and report.
Inpatient NTAP code – XXE2X19 – up to $1023.75 add onto the DRG payment for a HF related inpatient stay when EchoGo HF is used.
This is a significant step forward in the echo reimbursement landscape and should enable the wider adoption of the technology.
Echo AI Reimbursement Landscape diagram.
Growing Space
The reimbursement code breakthrough is also likely to bring others into exciting space, where many of our fellow AI EchoDx innovators are already making great progress.
InVision, co-founded by David Ouyang, a prolific publisher in the Echo AI space,11 has just announced a breakthrough designation for an amyloidosis screening model. If they can achieve FDA clearance, they could be the next entrant into the field. Another likely new entrant is iCardio.ai, which has recently published a paper on automated detection of aortic stenosis in the Journal of the American Society of Echocardiography (JASE).12
World of potential
AI EchoDx has the potential to turn the current, antiquated echo pipeline on its head. Even with the AI advancements, we still have to capture between 50 and 100 image clips, and make just as many measurements, before subsequently trying to piece together the diagnosis.
With the creation of AI EchoDx, you could imagine a future in which diagnosis could come at the point of the acquisition of a single image. This would allow us to tailor imaging to the patient in front of us, taking fewer, more specific measurements, according to the diagnostic recommendation.
Not only would this enhance speed and precision, but it would increase throughput, more accurately diagnose disease, and get more patients on the therapies they need to avoid hospitalization.
The trajectory of growth in AI EchoDx, however, will depend on our ability, as innovators, to prove the value of our solutions.
Isaac Kohane, editor-in-chief for NEJM AI, recently written that there is an “urgent need for randomized controlled trials of AI applications in medicine”. And I couldn’t agree more.
Isaac Kohane, editor in chief, NEJM AI, Injecting Artificial Intelligence into Medicine.13
Ultromics will have the read out from our EchoGo Pro RCT (PROTEUS) in the next few months, likely the first prospective RCT in the AI EchoDx space, and we recently published the protocol in the AHJ.14 We have further RCTs planned for EchoGo HF and EchoGo Amyloidosis.
In combination with prospective RCTs, we have found it of critical importance to team up with early adopter KOLs willing to cautiously adopt, implement and stress test the clinical utility of AI algorithms. The team at Northwestern including Drs Akhil Narang, Sanjiv Shah, Jim Thomas and Faraz Ahmad are some of the leaders in the field in thinking through how to adopt AI models into clinical practice. The combination of prospective studies and partnering with early adopter KOLs serves as a good recipe for other companies wanting to enter this space.
In summary, 10 years in, 2 FDA clearances, 2 new reimbursement codes and its first prospective RCT, I believe 2024 is going to be the year we see the clinical application of AI EchoDx take-off.
References:
- Borlaug BA, Sharma K, Shah SJ, Ho JE. Heart Failure With Preserved Ejection Fraction: JACC Scientific Statement. J Am Coll Cardiol. 2023;81(18):1810-1834.
- Upton R, Leeson P. Image-Based Diagnostic Systems. Published online June 17, 2021.
- Shah SJ, Katz DH, Selvaraj S, et al. Phenomapping for novel classification of heart failure with preserved ejection fraction. Circulation. 2015;131(3):269-279.
- Narula S, Shameer K, Salem Omar AM, Dudley JT, Sengupta PP. Machine-Learning Algorithms to Automate Morphological and Functional Assessments in 2D Echocardiography. J Am Coll Cardiol. 2016;68(21):2287-2295.
- Upton R, Mumith A, Beqiri A, et al. Automated Echocardiographic Detection of Severe Coronary Artery Disease Using Artificial Intelligence. JACC Cardiovasc Imaging. 2022;15(5):715-727.
- P. Akerman P, Mihaela Porumb P, Christopher G. Scott M, et a Automated Echocardiographic Detection of Heart Failure With Preserved Ejection Fraction Using Artificial Intelligence. JACC: Advances. 2023;2(6):100452.
- Anker SD, Butler J, Filippatos G, et al. Empagliflozin in Heart Failure with a Preserved Ejection Fraction. New England Journal of Medicine. 2021;385(16):1451-1461.
- Maurer MS, Schwartz JH, Gundapaneni B, et al. Tafamidis Treatment for Patients with Transthyretin Amyloid Cardiomyopathy. New England Journal of Medicine. 2018;379(11):1007-1016.
- González-López E, Gallego-Delgado M, Guzzo-Merello G, et al. Wild-type transthyretin amyloidosis as a cause of heart failure with preserved ejection fraction. Eur Heart J. 2015;36(38):2585-2594.
- Heidenreich PA, Albert NM, Allen LA, et al. Forecasting the impact of heart failure in the United States: a policy statement from the American Heart Association. Circ Heart Fail. 2013;6(3):606-619.
- Ouyang D, He B, Ghorbani A, et al. Video-based AI for beat-to-beat assessment of cardiac function. Nature 2020 580:7802. 2020;580(7802):252-256.
- Wessler BS, Huang Z, Long GM, et al. Automated Detection of Aortic Stenosis Using Machine Learning. J Am Soc Echocardiogr. 2023;36(4):411-420.
- Kohane IS. Injecting Artificial Intelligence into Medicine. NEJM AI. 2023;1(1). doi:10.1056/AIE2300197
- Woodward G, Bajre M, Bhattacharyya S, et al. PROTEUS Study: A Prospective Randomized Controlled Trial Evaluating the Use of Artificial Intelligence in Stress Echocardiography. Am Heart J. 2023;263:123-132.
Curious about upcoming research and innovation?
Sign up to hear about the latest news.

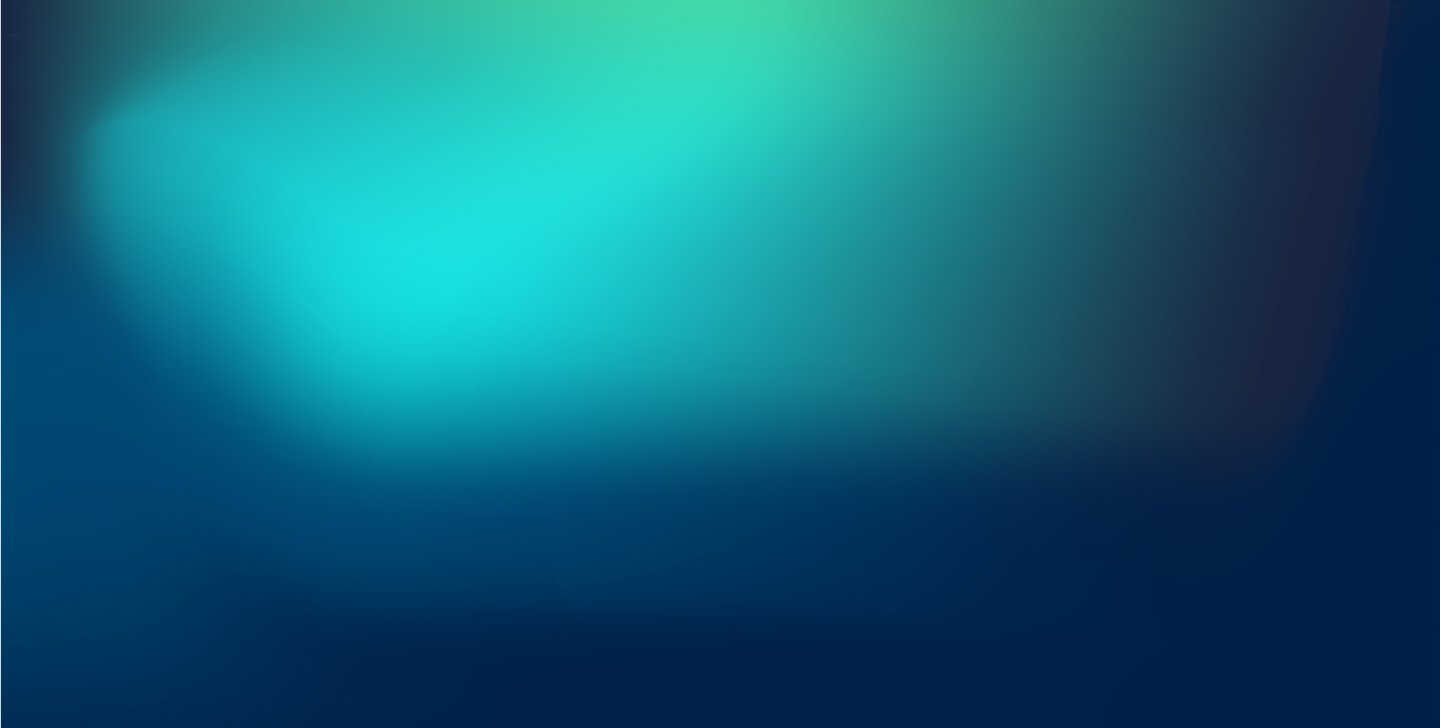