Jeremy A. Slivnick, Jorge Oliveira, Arian Beqiri, Amit R. Patel, Vidhushei Yogeswaran, Richard K Cheng, James N Kirkpatrick, Tetsuji Kitano, Masaaki Takeuchi, Karima Addetia, Gary Woodward, Roberto M. Lang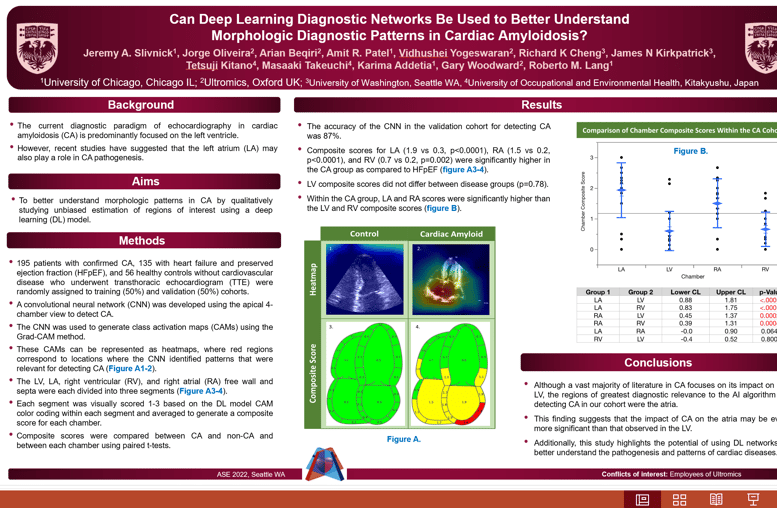
See full-size poster
Background
- The current diagnostic paradigm of echocardiography in cardiac amyloidosis (CA) is predominantly focused on the left ventricle.
- However, recent studies have suggested that the left atrium (LA) may also play a role in CA pathogenesis.
Methods
- 195 patients with confirmed CA, 135 with heart failure and preserved ejection fraction (HFpEF), and 56 healthy controls without cardiovascular disease who underwent transthoracic echocardiogram (TTE) were randomly assigned to training (50%) and validation (50%) cohorts.
- A convolutional neural network (CNN) was developed using the apical 4-chamber view to detect CA.
- The CNN was used to generate class activation maps (CAMs) using the Grad-CAM method.
- These CAMs can be represented as heatmaps, where red regions correspond to locations where the CNN identified patterns that were relevant for detecting CA.
- The LV, LA, right ventricular (RV), and right atrial (RA) free wall and septa were each divided into three segments.
- Each segment was visually scored 1-3 based on the DL model CAM color coding within each segment and averaged to generate a composite score for each chamber.
- Composite scores were compared between CA and non-CA and between each chamber using paired t-tests.
Results
- The accuracy of the CNN in the validation cohort for detecting CA was 87%.
- Composite scores for LA (1.9 vs 0.3, p<0.0001), RA (1.5 vs 0.2, p<0.0001), and RV (0.7 vs 0.2, p=0.002) were significantly higher in the CA group as compared to HFpEF.
- LV composite scores did not differ between disease groups (p=0.78).
- Within the CA group, LA and RA scores were significantly higher than the LV and RV composite scores.
Conclusion
- Although a vast majority of literature in CA focuses on its impact on the LV, the regions of greatest diagnostic relevance to the AI algorithm for detecting CA in our cohort were the atria.
- This finding suggests that the impact of CA on the atria may be even more significant than that observed in the LV.
- Additionally, this study highlights the potential of using DL networks to better understand the pathogenesis and patterns of cardiac diseases.
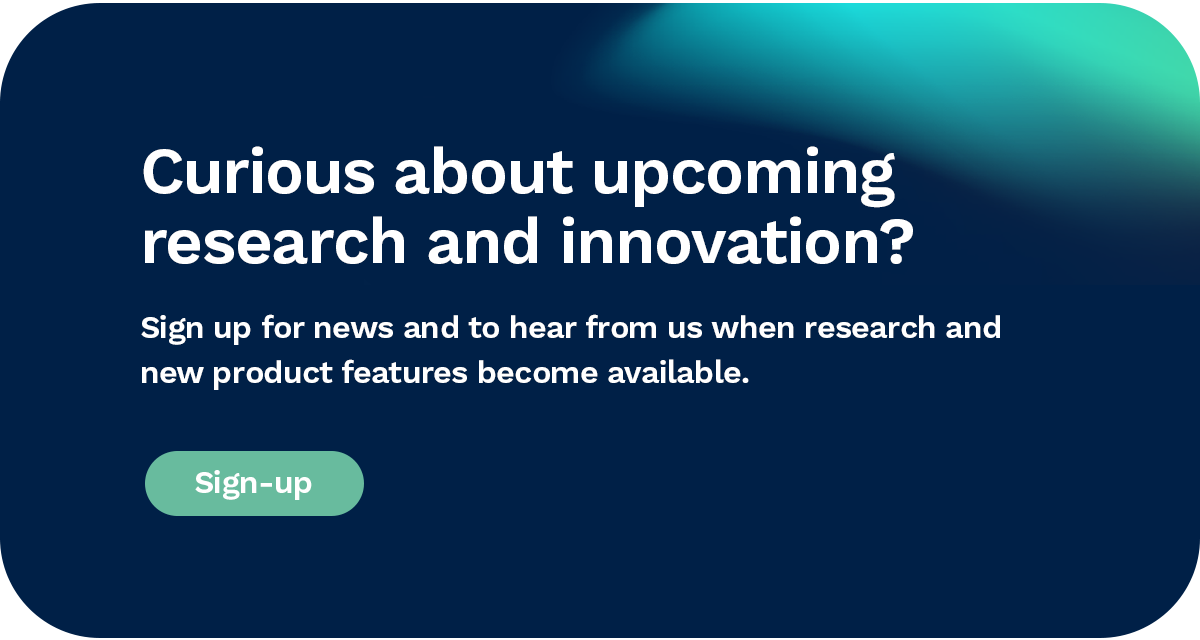